Table of Contents
In a previous blog, we discussed the concept of collective defense and explored its potential inclusion in machine learning practices to enhance security. In this blog, we will delve into how Lumu takes advantage of transfer learning and online learning techniques, combined with collective defense and data sharing, to improve the performance of its cybersecurity defense against various types of attacks. Additionally, we will provide a general overview of how deep learning works and highlight how Lumu utilizes these advanced techniques to bolster its clients’ cybersecurity response.
What Deep Learning Models Does Lumu Develop?
First, Deep Learning (DL), a subset of artificial intelligence, is exceptionally well-suited for handling patterns that contain a wealth of information. By utilizing deep neural networks, this approach can effectively process and analyze large volumes of data, enabling the discovery of intricate patterns and extracting meaningful insights. Whether it is images, text, or other forms of complex data, deep learning excels at capturing the richness and complexity within the patterns, allowing for accurate and comprehensive analysis. This remarkable ability to handle information-rich patterns has propelled deep learning to achieve remarkable breakthroughs across various domains, making it an invaluable tool in the field of artificial intelligence.
In this way, Lumu develops deep learning models tailored to enhance cybersecurity defenses. These models are designed to provide users with insights into the probability of potential attacks. With a vast amount of data gathered from the network behavior of all their clients, Lumu has established a robust foundation for their neural networks. This extensive dataset enables neural networks to effectively learn and adapt to emerging threats. Lumu leverages the power of transfer learning and online learning techniques to continually improve the performance of their models, ensuring a proactive and accurate assessment of the cybersecurity landscape for their clients. By combining these advanced learning methods with their rich dataset, Lumu empowers users with valuable insights and a heightened ability to protect their networks from potential attacks.
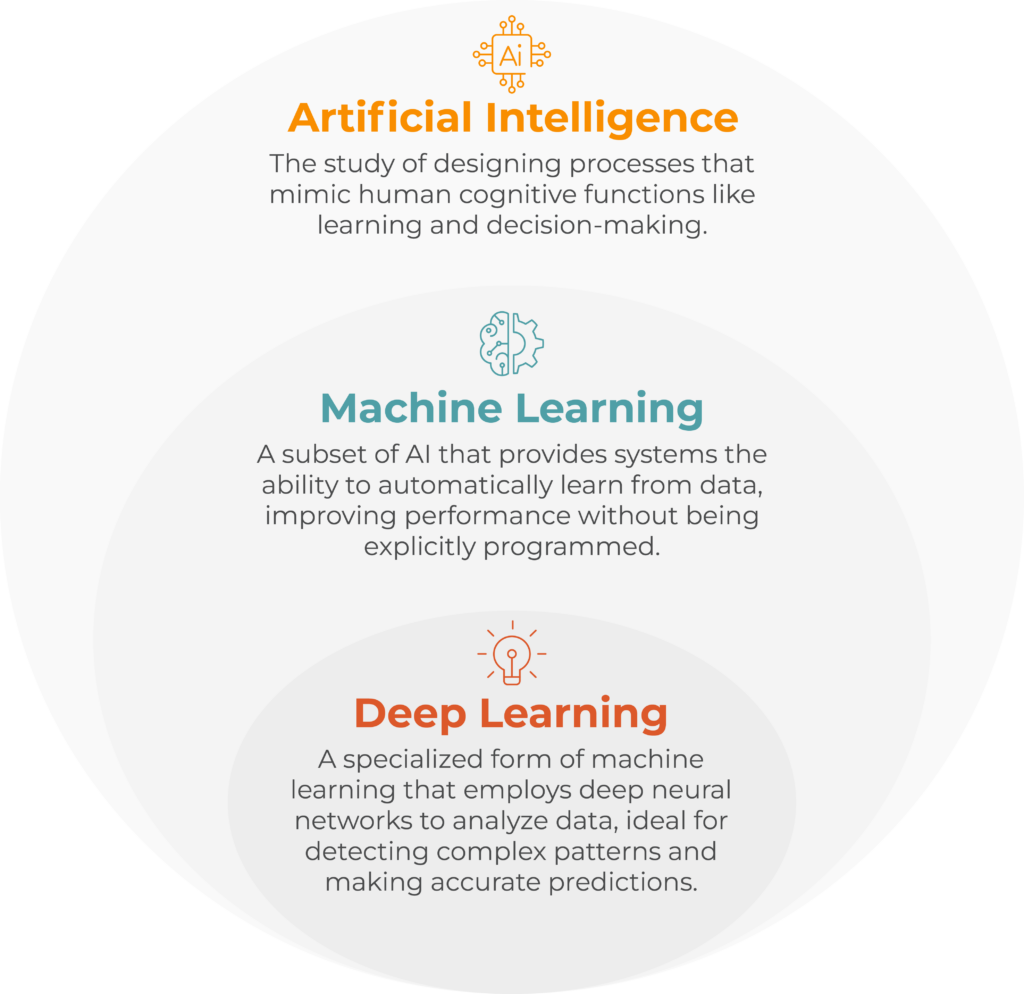
Why Do Transfer Learning and Online Learning Let Lumu Take Advantage of Collective Defense?
First, let’s define what transfer and online learning are. Transfer learning is a machine learning technique that involves applying knowledge gained from solving one problem to a different but related problem. This approach utilizes a pre-trained model, which has been trained on a large dataset, as a starting point for a new task. Instead of training a model from scratch, the pre-trained model’s knowledge and learned features are transferred, enabling faster and more accurate learning on the new task. Transfer learning is beneficial in situations with limited data and reduces the computational resources required for training.
On the other hand, Online Learning is an approach where a model is updated continuously as new data arrives. Unlike batch learning, where a fixed dataset is used for training, online learning allows the model to adapt to changes and learn from new data points in real time. This method is particularly useful in dynamic environments where the data distribution or the target task may change over time. By updating the model incrementally, online learning enables it to be more responsive to new information and facilitates continuous improvement without retraining the entire model. It finds applications in scenarios such as recommendation systems, fraud detection, and adaptive control systems.
Online Learning vs Transfer Learning
Online Learning | Transfer Learning | |
General Description | A machine learning approach that continuously updates the model as new data arrives, ideal for dynamic and real-time scenarios. | A technique that leverages a pre-trained model to accelerate learning for new but related tasks, useful when data or resources are limited. |
Data Requirements | Real-time, incremental | Pre-trained model on large dataset |
Training Method | Continuous updates | Initial training, then fine-tuning |
Adaptability | Adapts to new data in real-time | Adapts to new, but related, tasks |
Use Cases | Dynamic environments | Limited data scenarios |
Computational Requirements | Lower for each update | Varies, but often high initially |
Speed | Fast updates | Faster learning on new tasks |
In this way, Lumu utilizes Transfer Learning by deploying pre-trained models for new accounts. These pre-trained models have already learned from a vast amount of data gathered from various networks, including those of existing clients. By starting with these pre-trained models, Lumu can quickly initialize the security framework for new accounts and benefit from the knowledge acquired from previous experiences. This approach significantly reduces the time and resources required to develop effective cybersecurity models from scratch.
Secondly, Online Learning plays a crucial role in fine-tuning the pre-trained models to adapt to the unique characteristics of each network. As new data becomes available from the network behavior of clients, Lumu applies Online Learning techniques to continuously update and refine the models. This iterative learning process allows the models to learn from real-time insights and adapt to the evolving threat landscape. By actively incorporating new data and feedback, Lumu’s models become more accurate and efficient in detecting and mitigating cybersecurity attacks.
The combination of Transfer Learning and Online Learning enables Lumu to benefit from Collective Defense. As Lumu gathers data from the network behavior of all its clients, it accumulates a comprehensive understanding of various attack patterns and trends. This shared knowledge and insights acquired through Collective Defense enhance the effectiveness and accuracy of the models. Furthermore, as Lumu fine-tunes the models using Online Learning techniques, they continually improve their ability to identify emerging threats and provide proactive cybersecurity responses.
Conclusion and Final Thoughts
Recognizing the power and importance of collective defense in cybersecurity, Lumu harnesses its clients’ combined knowledge and leverages artificial intelligence’s capabilities to create and enhance machine learning models. These models accurately predict the probability of cybersecurity attacks. Lumu’s unique approach involves gathering clients’ information and incorporating it into their models, eliminating the need for a large historical dataset specific to each new client and saving time on training and model refinement.
By strategically utilizing Transfer Learning and Online Learning, Lumu establishes a robust and adaptive security framework. Leveraging pre-trained models enables quick initialization of security measures for new accounts, benefiting from accumulated collective knowledge. This approach ensures faster and more efficient deployment of cybersecurity measures.
Through Online Learning, Lumu keeps its models up-to-date and responsive to evolving threats. Continuous refinement and fine-tuning using real-time client network data enable effective detection and mitigation of emerging cybersecurity risks. This iterative learning process enhances overall client protection.
Incorporating Transfer Learning and Online Learning maximizes the benefits of Collective Defense for Lumu. The shared knowledge and insights gained from analyzing the network behavior of all clients improve model accuracy and effectiveness. This collective intelligence strengthens Lumu’s ability to detect patterns, identify anomalies, and proactively respond to potential attacks.
Ultimately, the combination of Transfer Learning, Online Learning, and Collective Defense empowers Lumu to continuously improve and enhance its cybersecurity capabilities. By leveraging shared knowledge, adapting to individual network characteristics, and staying ahead of emerging threats, Lumu ensures robust and proactive protection against cybersecurity attacks.
With this innovative approach, Lumu offers clients the benefits of collective defense and personalized insights. By leveraging artificial intelligence and incorporating client-specific data, Lumu provides accurate and tailored predictions, empowering organizations to proactively protect against cybersecurity threats. This collaborative and adaptive methodology ensures that Lumu remains at the forefront of cybersecurity defense, delivering effective and customized solutions to meet the unique requirements of each client.